Fairness of predictive models: an application to insurance markets
This project is led by Arthur Charpentier, Professor in the Department of Mathematics
at the University of Quebec in Montreal.
Duration of the project: 2023-2025
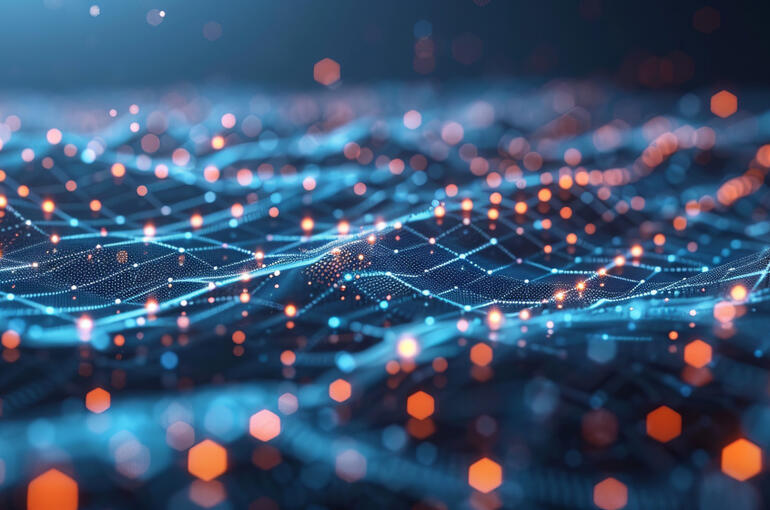
In the era of big data and barely interpretable machine learning techniques, ensuring fairness and non-discrimination in predictive models poses a significant challenge for the insurance industry. The differentiation between high-risk and low-risk policyholders lies at the core of this sector. However, with insurers gathering increasingly extensive data, it is becoming more complex to distinguish between justified pricing based on risk, and unfair discrimination based on sensitive factors. As Kranzberg's first law of technology states: "Technology is neither good nor bad; nor is it neutral." To prevent adverse effects, it is therefore imperative to accurately measure discrimination.
This project addresses biases within the automatic artificial intelligence algorithms utilized to determine optimal pricing in individual policies. Its aim is to mitigate or eliminate such biases, which could lead to inequities or discriminatory practices based on factors such as gender, race, religion, or origin in the coverage provided by insurers or reinsurers to policyholders.
The research topic has both theoretical significance, as it involves enhancing control over the "black box" nature of AI-based models, and practical importance, as it aims to reduce risks associated with discrimination and inequality. Given the increasing influence of social networks and the consequential reputational challenges faced by insurers and reinsurers, this issue is particularly relevant and timely.
Arthur Charpentier, PhD, Fellow of the French Institute of Actuaries*, is full professor at UQAM, Montreal, Canada, and Université de Rennes, in France. He is member of the editorial board of the Journal of Risk and Insurance, the ASTIN Bulletin, and Risks. He edited, a few years ago, Computational Actuarial Science with R (CRC), and more recently wrote Insurance, Biases, Discrimination and Fairness (Springer). He is also the former director of the Data Science for Actuaries program of the French Institute of Actuaries. He is a Louis Bachelier Fellow, and his recent work is about climate change and predictive modeling insurance, more specifically in the context of fairness and discrimination.
Alma mater: MSc in actuarial science ENSAE (1999, Paristech, France), MSc in mathematical economics Paris Dauphine (1999, PSL, France), PhD in applied mathematics KU Leuven (2006, Belgium), HDR* in applied mathematics Université de Rennes (2016, France).