Fairness of predictive models: an application to insurance markets | Mathematical Foundations of AI Day
Keynote presentation: Optimal transport and fairness of predictive models - September 2024
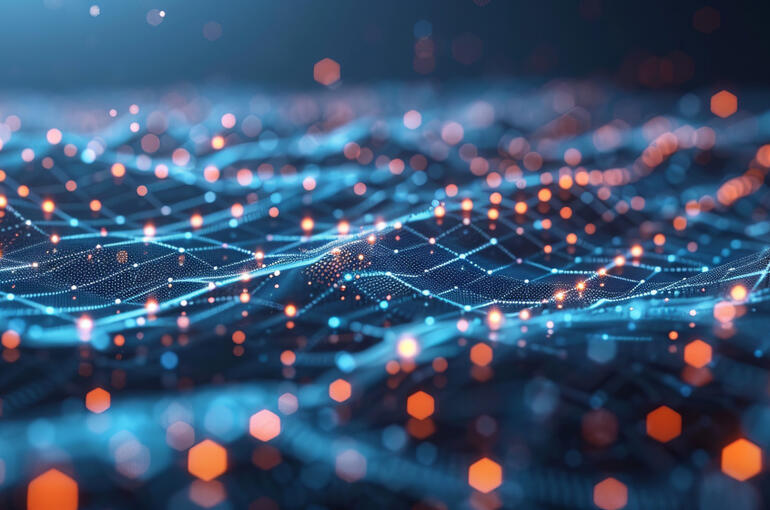
Led by Professor Arthur Charpentier from the University of Quebec in Montreal, this project focuses on addressing biases in automated artificial intelligence algorithms used to determine optimal pricing in individual insurance policies. The goal is to mitigate or eliminate these biases, which could result in inequities or discriminatory practices based on factors like gender, race, religion, or origin in the coverage offered by insurers or reinsurers to policyholders.
In September 2024, Professor Charpentier gave a keynote presentation titled “Optimal transport and fairness of predictive models” at an annual workshop in Paris dedicated to the Mathematical Foundations of AI, organized by the DATAIA Institute and SCAI (Sorbonne Center for Artificial Intelligence). The introduction below is taken from his blog https://freakonometrics.hypotheses.org/75138:
In this talk, we present two complementary approaches to addressing fairness in algorithmic decision-making through the lens of counterfactual reasoning and optimal transport, both in individual and group fairness. First, we introduce a novel method that links two existing counterfactual approaches: causal graph-based adaptations (Plečko and Meinshausen, 2020) and optimal transport (De Lara et al., 2024). By extending “Knothe’s rearrangement” (Bonnotte, 2013) and “triangular transport” (Zech and Marzouk, 2022) to probabilistic graphical models, we propose a new group framework, termed sequential transport, which we apply to the problem of individual fairness. Theoretical foundations are established, followed by numerical demonstrations on synthetic and real datasets. Building on this, we extend the discussion to algorithmic fairness in the presence of multiple sensitive attributes. While traditional fairness frameworks focus on eliminating bias with respect to a single sensitive variable, their effectiveness diminishes with multiple sensitive characteristics. To address this, we propose a sequential fairness framework based on multi-marginal Wasserstein barycenters, generalizing Strong Demographic Parity to handle multiple sensitive features. Our method provides a closed-form solution for the optimal, sequentially fair predictor, enabling interpretation of correlations between sensitive attributes. Furthermore, we introduce an approximate fairness framework that balances risk and unfairness, allowing for prioritization of fairness across specific attributes. Both approaches are supported by comprehensive numerical experiments on synthetic and real-world datasets, showcasing the practical efficacy of these methods in promoting fair decision-making. Together, they provide a robust framework for addressing fairness in complex, multi-attribute settings while preserving interpretability and flexibility.