Fairness of predictive models: an application to insurance markets
Recent events: Presentation at ACP, KU Leuven and workshop at IME in Chicago - July 2024
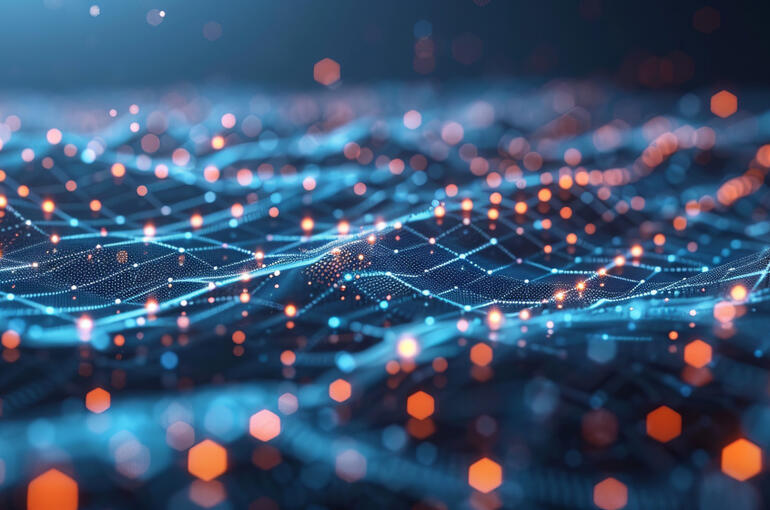
Led by Professor Arthur Charpentier from the University of Quebec in Montreal, this project focuses on addressing biases in automated artificial intelligence algorithms used to determine optimal pricing in individual insurance policies. The goal is to mitigate or eliminate these biases, which could result in inequities or discriminatory practices based on factors like gender, race, religion, or origin in the coverage offered by insurers or reinsurers to policyholders.
In June and July 2024, Professor Charpentier spoke at two events linked to the project, giving a presentation in Belgium and leading a workshop in the USA.
- As part of the Actuarial Contact Program (ACP) held at KU Leuven in June, he gave a presentation titled “From contemplative to predictive modeling in actuarial science and risk management”. The introduction below is taken from his blog Freakonometrics (hypotheses.org):
It is usually claimed that actuaries build 'predictive models' but most of the time, what they consider would be simply 'contemplative modeling', in the sense that they use past information and hope that the future will be more or less the same (corresponding to the idea of generalization in machine learning). In the context of climate change (but also when modeling insurance market competition) it is not the case, data used to train models do not have the same distribution as the one we will have in the future.
At the 27th Congress on Insurance: Mathematics and Economics (IME) held in Chicago in July, he led a workshop on decentralized insurance and risk sharing, titled "Collaborative insurance, unfairness, and discrimination". The introduction below is taken from Freakonometrics (hypotheses.org):
In this course, the researchers will get back to mathematical properties of risk sharing on networks, with reciprocal contracts. They will discuss conditions about stochastic dominance, proving that policyholders might have interest in sharing risks with “friends”. Then, they will try to address fairness issues, for such risk sharing mechanisms. If fairness has been recently intensively studied, either through group or individual fairness, there are yet not much literature about fairness on networks. It is important to address those issues since perceived discrimination is usually associated with networks. We will see why the topology of the network is important, both to design peer-to-peer schemes to share risks, but also to see if perceived discrimination is associated with global disparate treatment.