SCOR Chair on Mortality Research | Publication of two research articles
Articles published in the American Journal of Epidemiology and Demographic Research - September 2024
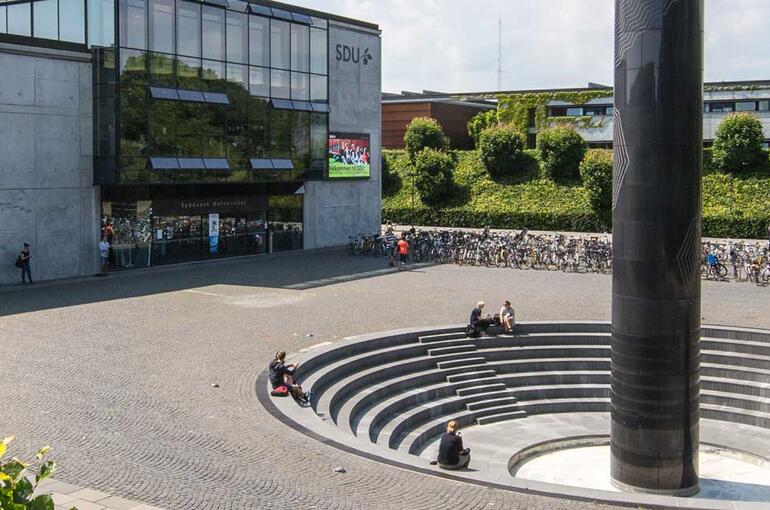
The SCOR Chair on Mortality Research is led by Marie-Pier Bergeron-Boucher, Associate Professor at the Interdisciplinary Center on Population Dynamics (CPop) at the University of Southern Denmark. It aims to initiate research in the fields of demography and actuarial science applied to the analysis of mortality and longevity, focusing on three main topics:
Analyzing multi-morbidities and causes of death,
Forecasting mortality,
Developing demographic models to study changes in mortality.
Two articles by the Chair’s researchers were recently published in the American Journal of Epidemiology and Demographic Research:
“Month-to-month all-cause mortality forecasting: a method allowing for changes in seasonal patterns”
Ainhoa-Elena Léger and Silvia Rizzi, American Journal of Epidemiology
Abstract
Forecasting of seasonal mortality patterns can provide useful information for planning health-care demand and capacity. Timely mortality forecasts are needed during severe winter spikes and/or pandemic waves to guide policy-making and public health decisions. In this article, we propose a flexible method for forecasting all-cause mortality in real time considering short-term changes in seasonal patterns within an epidemiologic year. All-cause mortality data have the advantage of being available with less delay than cause-specific mortality data. In this study, we use all-cause monthly death counts obtained from the national statistical offices of Denmark, France, Spain, and Sweden from epidemic seasons 2012-2013 through 2021-2022 to demonstrate the performance of the proposed approach. The method forecasts deaths 1 month ahead, based on their expected ratio to the next month. Prediction intervals are obtained via bootstrapping. The forecasts accurately predict the winter mortality peaks before the COVID-19 pandemic. Although the method predicts mortality less accurately during the first wave of the COVID-19 pandemic, it captures the aspects of later waves better than other traditional methods. The method is attractive for health researchers and governmental offices for aiding public health responses because it uses minimal input data, makes simple and intuitive assumptions, and provides accurate forecasts both during seasonal influenza epidemics and during novel virus pandemics.
“Makeham mortality models as mixtures: Advancing mortality estimations through competing risks frameworks”
Silvio C. Patricio and Trifon I. Missov, Demographic Research
Abstract
BACKGROUND
The Makeham term serves as a fundamental component in mortality modeling, offering a constant additive hazard that accounts for background mortality factors usually unrelated to the aging process. This term, widely employed in mortality analysis, provides a crucial mechanism for capturing mortality risks unrelated to age-related deterioration.
OBJECTIVE
The objective of this paper is to explore how Makeham models, which are widely used for studying mortality, can be understood and analyzed within the context of competing risks. The paper seeks to provide insights into the mathematical properties, interpretation, and applicability of Makeham models in modeling age-dependent and age-independent mortality risks. Additionally, the paper aims to demonstrate formally that competing-risk models can be represented as mixture models, thereby facilitating a deeper understanding of risk-specific mortality dynamics.
CONTRIBUTION
Expressing competing-risk models as mixtures aids identifying the overall and agespecific share of deaths according to each of the competing risks. In particular, Makeham mortality models, when represented as mixtures, provide, first, a semantically and computationally convenient platform to disentangle age-dependent from age-independent mortality, and second, a straightforward specification that can easily be extended to account for unobserved heterogeneity. By expressing Makeham models as a convex combination of probability distributions, we are able to estimate the age-profile of age-independent mortality, especially at the oldest ages, at which we intuitively assume that most deaths are age-dependent (senescent). We are also able to estimate the senescent mortality component, which is the one to focus on when studying the aging process and its characteristics.